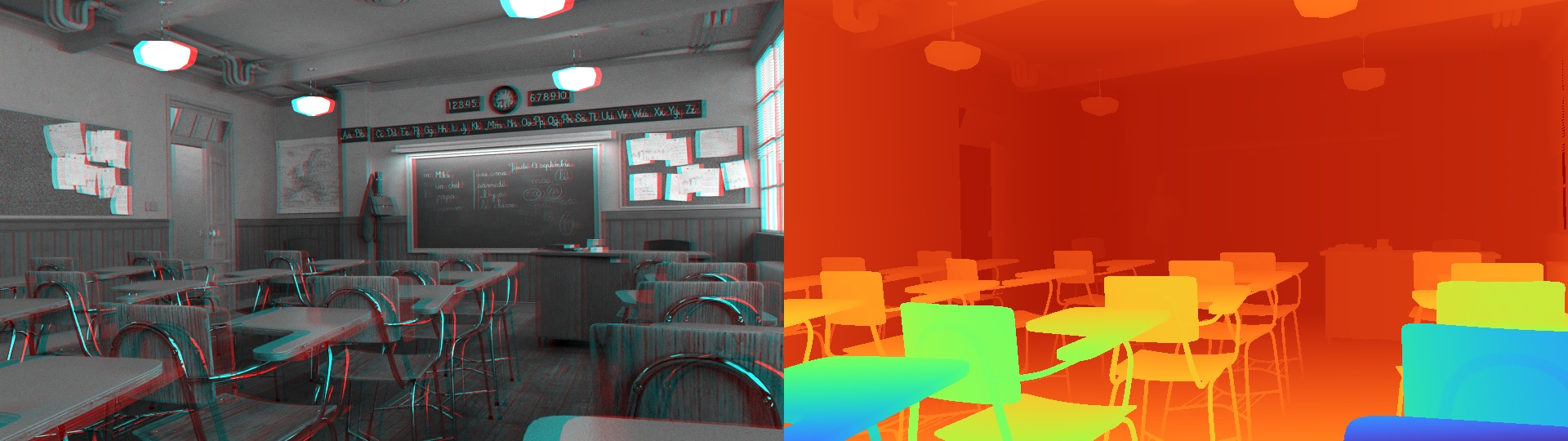
Logistics
You can download the raw material (hw3_2024.zip
) and the supplementary slides (hw3_supplementary_slides.pdf
) from KLMS.
To do
-
Code template in
code/
. -
Writeup template in
writeup/
. Writeup is important for this homework. You must answer the questions in the template with writing the description about your code. If you don’t submit the writeup or submit it as an empty, you will get 0 points for this homework.
Submission
-
Due: Friday, Nov. 8th 2024, 23:59
-
Do NOT change the file structure and
hw3_main.py
file. -
Use
create_submission_zip.py
to create a submission zip file. -
Rename your submission zip file to
hw3_studentID_name.zip
(ex: hw3_20241234_MinHKim.zip). -
The submission zip file must include your code and writeup (in pdf). Check your zip file includes successfully compiled writeup.
-
Submit your homework to KLMS.
-
An assignment after its original due date will be degraded 20% per day (see course introduction for detail).
-
Again, double-check your zip file uploaded on KLMS. We will not alert you immediately if your submission is empty or the wrong file.
QnA
-
Please use KLMS QnA board, and title of the post like: [hw3] question about … .
-
Note that TAs are trying to answer question ASAP, but it may take 1—2 day on weekend. Please post the question on weekday if possible.
-
TA in charge: Donggun Kim <dgkim1003@kaist.ac.kr>
Overview
You will implement codes for image rectification and a basic stereo algorithm. We provide some pairs of images and feature point sets from corresponding images. There are the 5 tasks with function names below.
-
(a) Bayer image interpolation. (
bayer_to_rgb_bilinear()
) -
(b) Find fundamental matrix using the normalized eight point algorithm. (
calculate_fundamental_matrix()
) -
(c) Rectify stereo images by applying homography. (
modify_rectification_matrices()
,transform_fundamental_matrix()
). -
(d) Make a cost volume using zero-mean NCC(normalized cross correlation) matching cost function for the two rectified images, then obtain disparity map from the cost volume after aggregate it with a box filter. (
compute_cost_volume()
,filter_cost_volume()
,estimate_disparity_map()
).
Forbidden functions
If you use following functions, you will get 0 score for this homework.
-
np.correlate()
,np.corrcoef()
-
cv2.cvtColor()
,cv2.demosaicing()
-
cv2.stereo*()
(e.g.,cv2,stereoBM()
,cv2.stereoRectify()
, …) -
cv2.get*Transform()
(e.g.,cv2.getPerspectiveTransform()
, …) -
cv2.findEssentialMat()
,cv2.findFundamentalMat()
,cv2.findHomography()
-
Any other pre-implemented functions to get direct result of main task.
Useful functions
-
np.linalg.inv()
,np.linalg.svd()
-
cv2.perspectiveTransform()
Code details
[NB] We will only use hw3_functions.py
from your submission for grading. To keep consistency with the grading environment, do NOT modify the main code hw3_main.py
and its function name and input variables. Note that only basic scientific libraries are installed in the grading machine (e.g. Numpy, Sicpy and OpenCV) with other commonly used Python libraries (e.g. tqdm, matplotlib). You are not allowed to use the following libraries in this homework: multiprocessing, CUDA, pytorch, JAX, tensorflow, etc., which can utilize process-level parallelism or GPU.
hw3_main.py
The main code starts by reading images and loading feature points from the data directory. Reading and loading codes are already implemented. The output of the main code will be saved in the result/
directory automatically. You need those output images for your writeup.
-
(a) Bilinear interpolated images:
bilinear_img*.png
-
(b) Images overlayed with feature points and their epipolar lines:
imgs_epipolar_overlay.png
-
(c) Rectified images (w/ features & epipolar lines overlay):
rectified_img*.png
,rectified_imgs_pair.png
,rectified_imgs_epipolar_overlay.png
/ Anaglyph after rectification:rectified_anaglyph
/ Values of fundamental matrices between rectified images:fundamental_matrix_rectified_imgs_*.txt
-
(d) Calculated disparity map, as color mapped plot
disparity_map.png
and raw datadisparity_map.npy
hw3_functions.py
Here you implement the functions. You have to implement 5 functions named:
-
(a)
bayer_to_rgb_bilinear
-
(b)
calculate_fundamental_matrix
-
(c)
modify_rectification_matrices
-
(c)
transform_fundamental_matrix
-
(d)
compute_cost_volume
-
(d)
filter_cost_volume
-
(d)
estimate_disparity_map
Also, there are some hyperparameters (WINDOW_SIZE
, MIN_DISPARITY
, MAX_DISPARITY
, AGG_FILTER_SIZE
) at the top of this code. You can modify these to get the best results (actually, you must). More details will be provided in the next Task details section.
utils.py
There are some utility functions. You can freely use the function here. Some of them are already used in main code.
-
homogenize
: Make nonhomogeneous coordinate vectors (or matrices/arrays obtained by stacking those vectors) by appending 1’s as last elements. -
normalize_points
: Center the image data at the origin, and scale it so the mean squared distance between the origin and the data points is 2 pixels. This function is already implemented and you do not need to work on it. -
image_overlay_line
,image_overlay_circle
,images_overlay_fundamental
,get_anaglyph
,draw_epipolar_overlayed_img
draw_stereo_rectified_img
,draw_disparity_map
: Functions to visualize your work. These functions are already used in main code. -
get_time_limit
: Compute the disparity map computing time limit of your machine based on some basic operations. See Task details section for the detail. -
compute_epe
: Computes the evaluation metric. We will use 2 type of metric, details in the next Task details section.
Task details
(a) Bayer image interpolation
-
TODO code:
bayer_to_rgb_bilinear
,
We provided some pairs of images (data/img1_bayer.png
and data/img2_bayer.png
) for the stereo algorithm. But, the images are RGGB Bayer patterned, so that you need to convert them into RGB color images first. There are many interpolation methods, but here we only cover bilinear interpolation. Note that you should implement Bayer to "RGB" (general), NOT "BGR" (which openCV uses). RGB to BGR conversion will be automatically done in the main code.
Raw image data obtained by cameras such as our data image have a Bayer pattern. Alternating pixels in each of these images store different color intensities. Several Bayer patterns exist, but our data images have RGGB patterns. This means four pixels in each 2*2 window in the image store Red, Green, Green, and Blue color intensity, respectively. The following figure represents RGGB Bayer patterns.
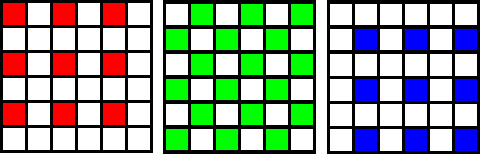
To implement Bayer image interpolation, you should first extract each channel from the raw image. Then, you will have three channels for the image, but each channel of the image has many missing values. The white blocks in the above figure mean missing pixels of each channel. 3/4 of the R and B channels and half of the G channel are missing. So, you should implement interpolation for each image channel.
For border pixels, you can handle it on your own. You can use zero padding, reflect padding, only use three neighboring pixels, … whatever you think is right. Just clarify your method in the writeup. i.e., state why you used that method. If your implementation of interpolation is correct in the non-border regions, you can get full credit. FYI, usually, border pixels matter less in a real camera since a few border pixels are unused after Bayer interpolation. (There are some sensors that do not use Bayer, except them.) That’s one reason for the difference in total pixels and effective pixels in a real camera. However, in this homework, your image size should not be changed, and you should think about how you can handle it.
(b) Calculate fundamental matrix
-
TODO code:
calculate_fundamental_matrix
From the given two sets of feature points, find the fundamental matrix using the normalized eight-point algorithm. Use the normalize_points
function to normalize points.
We have learned the definition and properties of fundamental matrices. After implementing Bayer image interpolation, you should implement the eight-point algorithm to find the fundamental matrix. Details for the eight-point algorithm is described in the supplementary slides in the provided zip file. You can study only the "Eight Point Algorithm: Implementation" slide to implement this task, but "Eight Point Algorithm: Proof" slides in the supplementary material will be helpful if you are interested in optimization methods and a detailed proof for the normalized eight-point algorithm.
Note that a fundamental matrix can convert an image point into its epipolar line on the opposite image. After implementing calculate_fundamental_matrix
, hw3_main.py
will generate an image file result/imgs_epipolar_overlay.png
, which is overlayed with the feature points and their epipolar lines. You can utilize this image to check your implementation qualitatively.
(c) Stereo rectification
After finding the fundamental matrix, you should rectify your cameras. It means that we need to reproject the input image planes onto the common plane parallel to the line between camera centers.
After the reprojection, the pixel motion will be horizontal. This reprojection is formed as two homography matrices(3*3) \(H\) and \(H'\), one for each input image reprojection. Those \(H\) and \(H'\) can be derived from the fundamental matrix and feature point sets. However, it requires many more steps than we covered in the lecture. Thus, finding \(H\) and \(H'\) is done by the OpenCV native function cv2.stereoRectifyUncalibrated()
, and you do not need to work on that part. What you need to do is the following two tasks.
i) Rectification without cropping
-
TODO code:
modify_rectification_matrices
Once you got two homography matrices \(H\) and \(H'\), you can actually apply those transformations to the images. However, these \(H\) and \(H'\) obtained by cv2.stereoRectifyUncalibrated()
will produce cropped images. There are many ways to get rectified images without cropping, but here we give one choice: modifying the homographies. In modify_rectification_matrices
, you should modify \(H\) and \(H'\) to \(H_{\mathrm{mod}}\) and \(H_{\mathrm{mod}}'\), which also indicate rectification and do not yield cropping. Note that cv2.perspectiveTransform()
may be useful.
ii) Rectification constraint for fundamental matrices
-
TODO code:
transform_fundamental_matrix
Now, we will validate the mathematical constraint of rectification that should be satisfied. First, you have to recalculate the fundamental matrix between rectified images. You need to implement transform_fundamental_matrix
to compute the fundamental matrix between rectified images using the original fundamental matrix and \(H\) and \(H'\). At the end of the task (c), The output fundamental matrix between rectified images will be written in the result/fundamental_matrix_rectified_imgs_original.txt
. You should report this matrix value in your writeup. You may also find some constraints of the resulting fundamental matrix. Also, write the constraint that fundamental matrices between rectified images satisfy in your writeup.
After that, you will validate your modification implemented in modify_rectification_matrices
. The result using \(H_{\mathrm{mod}}\) and \(H_{\mathrm{mod}}'\) will be written in the result/fundamental_matrix_rectified_imgs_modified.txt
.
You sould also report this to the writeup and write the relationship between fundamental_matrix_rectified_imgs_original
.
(d) Calculate disparity map
-
TODO code:
compute_cost_volume
,filter_cost_volume
,estimate_disparity_map
It is possible to estimate a disparity map from your rectified images. But for better evaluation of a disparity map estimation itself, we provide perfect rectified images from a calibrated camera (data/img3.png
and data/img4.png
) and ground truth disparity map (data/img3_disp.npy
). The data reading part has already been implemented, so you don’t need to work on it. And, of course, do NOT utilize ground truth disparity to calculate your disparity map. If we find this kind of cheating, you will get 0 points in this homework, whatever you did.
The final goal of this task is to get the disparity map of first image (left image, data/img3.png
). In other words, for such point \((x,y)\) in first image, the corresponding point in second image (right image, data/img4.png
) is given as:
where \(\mathbf{d}(x, y)\) is disparity map of first image. (Hint: In general binocular stereo setup and the rectified left/right images, \(\mathbf{d}(x, y)\) of left image might have negative values. i.e., maximum possible disparity is 0. Think why it happens.) Since this process takes a long time, it is recommended to print the progress of computation log to check your code is running (e.g., print iteration numbers, using the tqdm
library or any other methods).
Before starting, there are some hyperparameters such as NCC window size, minimum and maximum disparities, and cost aggregation window size. Try various hyperparameter values and search for good values on your own. Provide your findings in the writeup. For the window size parameters, you are allowed assume it will be only the odd numbers.
In addition, you may see some numerical computation warnings for this task, such as zero-division or overflow. Make sure your code does NOT produce such numerical warnings. If your result contains nan (not a number) or inf (infinity), it will be reported as 0 points on the evaluation.
i) Cost volume computation
-
TODO code:
compute_cost_volume
Start by constructing a 3D cost volume of H x W x D, where H is the image’s height, W is the image’s width, and D is the number of disparities related to the minimum and maximum disparities. Then, you need to fill out the value of cost by calculating zero-mean NCC between a block at \((x, y)\) and a block at \((x + d, y)\). You may choose your own window size, and D. Larger window size gives less noisy results but suffers from edge bleeding. Test the performance of the various sizes of block windows. You must implement zero-mean NCC that we learned in class. If you implement zero-mean NCC correctly, your disparity map result (not cost volume) should be almost consistent when changing the image’s brightness scale and offset by an arbitrary value. Note that this arbitrary value will be changed on the grading, so do NOT utilize those values. Replace self.img4_gray
to self.img4_gray_transformed
in the hw3_main.py
Line 132 to test this. But note that passing this test does not ensure your correctness of zero-mean NCC. It is a sufficient condition, not a necessary and sufficient condition.
ii) Cost volume filtering:
-
TODO code:
filter_cost_volume
The initial cost volume contains a lot of noise due to inaccurate matching values. Cost aggregation is a process for removing those noisy values by smoothing them out. A naive method for cost aggregation, which you have to implement, is applying a box filter for each cost plane of a specific disparity \(d\). There can be many other sophisticated methods for cost aggregation. If you implement one (other than box filter) and explain the difference in your writeup, you can get +5 extra points.
iii) Estimate disparity
-
TODO code:
estimate_disparity_map
The final step is deciding the winning disparity of each pixel by finding the optimal cost value at \((x, y)\). You can also estimate sub-pixel disparity (you learned in class) from the cost volume, not just select the winning disparity. If you implement this and explain it in the writeup, you can get +10 extra points. NB, Try sub-pixel disparity estimation after successfully implementing the disparity map computation.
iv) Disparity map evaluation
Your whole function for the disparity map estimation should be run within the time limit computed by the get_time_limit()
function. Otherwise, you will not get a score for the task (d). Make it as efficient as you can. In addition, you are not allowed to downsample the image or cost volume to speed up your code. If you do this kind of cheating, your code will regarded as exceeding the time limit. i.e., you will get 0 points for this task (d).
You must also write the calculated time limit from get_time_limit()
of your machine, the running time on your machine, and your machine information (CPU info, …) in the writeup. The get_time_limit()
function will run only once, and save the result at data/timelimit.txt
and use it for the future. If you want to re-compute the time limit, just delete the data/timelimit.txt
file.
FYI, solution code based on the naive plane sweep algorithm to generate the disparity map with full credit runs about 1/3 of the time limit (around 10s in TA’s PC). Note that this is not the best. It can be further more efficient, even runs within 1/30 of the time limit (around 1s in TA’s PC) with the same quality disparity map.
We will use two metrics to evaluate your disparity map. EPE (End point error) and Bad pixel ratio. The evaluation function is already implemented in utils.py
, and used in main code, so you don’t need to do additional things. EPE measures the root mean squared error (RMSE) between the estimated disparity map and the ground truth disparity map. Bad pixel ratio measures the ratio of the pixels which has EPE higher than such threshold. We use 3px as a threshold to decide whether it is a bad pixel. There are example evaluation results in the supplementary slide.
Writeup
Again, writeup is important for this homework. If you don’t submit the writeup or submit it as an empty template, you will receive 0 points for this homework, whatever you did. Describe your process and algorithm, show your results, describe any extra credit, and tell us any other information you feel is relevant. We provide you with a LaTeX template. You MUST answer the questions in the template and write a description of your code. If any of them are missing, you will get no score for the corresponding task whatever you did in the code. Please compile it into a PDF and submit it with your code.
Rubric and Extra Credit
Total 100pts
-
[+10 pts] Implement bilinear interpolation to get RGB imgaes from RGGB bayer images. (
bayer_to_rgb_bilinear
) -
[+15 pts] Find the fundamental matrix from given two feature point sets. (
calculate_fundamental_matrix
) -
[+10 pts] Rectify stereo images by applying homography.
-
[+5 pts] Implementing modification in correct way. (
modify_rectification_matrices
) -
[+5 pts] Implementing the computaion to get the fundamental matrix between transformed images. (
transform_fundamental_matrix
)
-
-
[+35 pts] Disparity map computation. 0 pts if running time >=
get_time_limit()
-
[+20 pts] EPE (End-point-error)
-
[20 pts] \(\mathsf{EPE}<2.5\)
-
[15 pts] \(2.5\le \mathsf{EPE}<3.0\)
-
[10 pts] \(3.0\le \mathsf{EPE}<4.0\)
-
[5 pts] \(4.0\le \mathsf{EPE}<5.0\)
-
[0 pts] \(5.0\le \mathsf{EPE}\)
-
-
[+15 pts] Bad pixel ratio
-
[15 pts] \(\mathsf{Bad\ pixel\ ratio}<25\%\)
-
[10 pts] \(25\%\le\mathsf{Bad\ pixel\ ratio}<30\%\)
-
[5 pts] \(30\%\le\mathsf{Bad\ pixel\ ratio}<40\%\)
-
[0 pts] \(40\%\le\mathsf{Bad\ pixel\ ratio}\)
-
-
-
[+30 pts] Writeup.
Extra credit
Total 20pts
-
[+5 pts] Implement any existing or sophisticated cost aggregation algorithm other than the box filter and explain in the writeup.
-
[+10 pts] Sub-pixel disparity interpolation and explain in the writeup. NB, Try this after you successfully implement disparity map estimation.
-
[+5 pts] For comprehensive comparisons, and describing detaild experiment descriptions in the writeup.
To get extra credits, you MUST describe BOTH the original version and the extra-credit version in the writeup. For code submission, you can submit only the extra credit-enabled code with parameters to switch between the original task and the extra credit task.
Credits
The original project description and code are made by James Hays based on a similar project by Derek Hoiem. The Python version of this project is revised by Dahyun Kang, Inseung Hwang, Donggun Kim, Jungwoo Kim, Shinyoung Yi and Min H. Kim.